A modern CRM system serves as the central nervous system for managing interactions and data related...
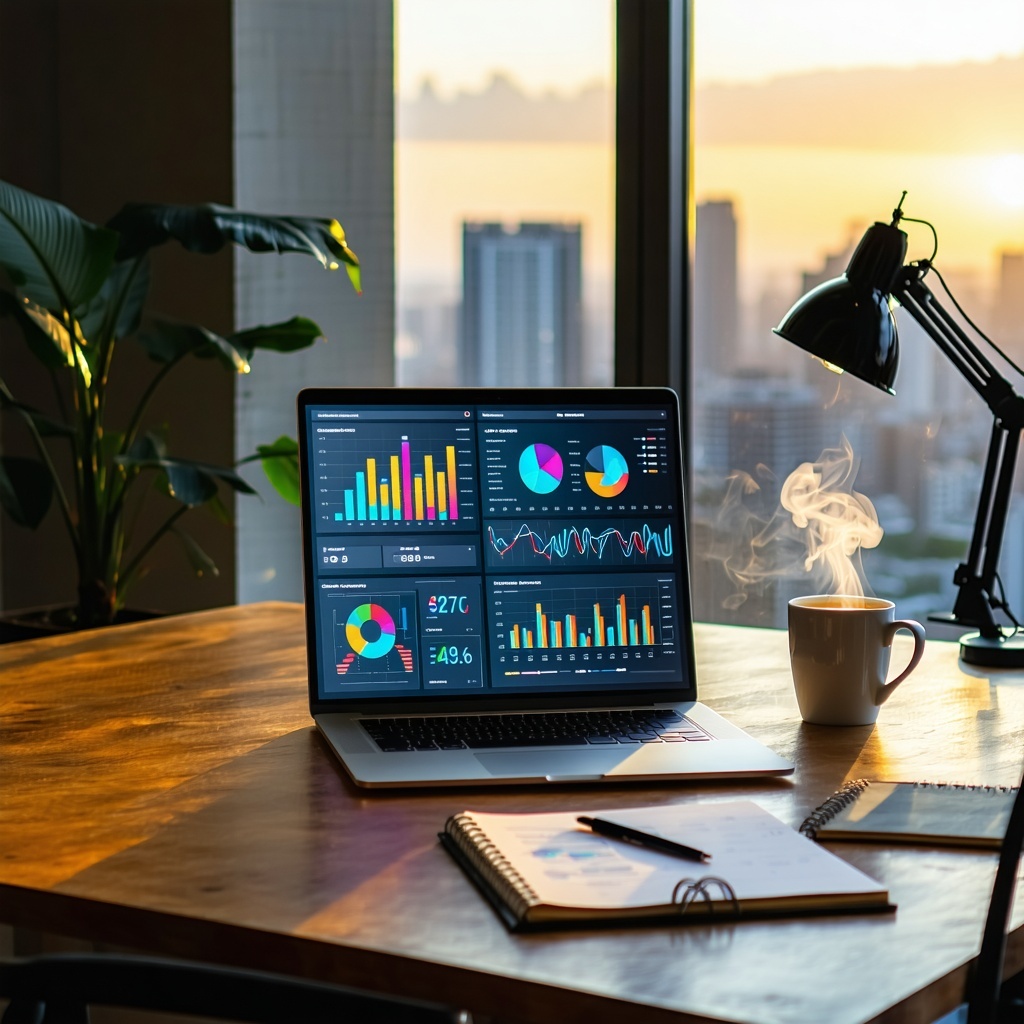
A holistic approach to assure the quality of your data
Data stored by business and organizations is the lifeblood of their operation and a key factor to their success. Data is a valuable asset and sometimes even a significant part of their property. It is a tool to understand and to serve their customers, the enabler for all marketing and sales actions, the key for financial management, the tool for operational excellence and for some business it is a part of their product. However, not all data is equally useful or reliable. Having a high quality of data is the safest way to ensure the validity and reliability of data use whether it is an ad hoc use or an analysis of any kind. Therefore, businesses should strive to collect, store, process, and analyze data that is not only sufficient in quantity but also superior in quality.
Data quality can be defined on the basis of the follow criteria, the basic characteristics of high quality data.
Sufficient, without missing values.
Meaningful for the context and objectives.
Coherent and non-contradictory.
Available and easy to access, retrieve, and use.
Reflecting the most recent changes and updates.
Ensuring high quality data requires a set of several specialized and sometimes difficult tasks falling under the the process of Data quality management. This is a complicated job requiring specialized people and tools but first and most important of all, a perpetual commitment for management and all basic stakeholders.
All those tasks may be grouped by defining four main processes or functions related to quality management:
This process involves setting the framework for data quality and defining all standards and criteria for data quality, such as accuracy, completeness, consistency, timeliness, etc. Data quality rules are the specific conditions or validations that data must meet to comply with the standards. This is a process that is usually made in house, with all stakeholders involved and with management involvement.
This process involves measuring and evaluating the current state of data quality using various methods and tools, such as data profiling, data cleansing, data auditing, etc. Data quality assessment can reveal the sources and types of data quality issues, providing recommendations for data quality improvement.
This is about actively improving data quality by correcting, enhancing, or deleting the data that does not meet the quality standards or rules. Data quality resolution can be done manually or automatically using various techniques and tools, such as data transformation, data enrichment, data matching, data deduplication, etc.
This process involves tracking and reporting the changes and trends in data quality over time using various metrics and indicators, such as data quality score, data quality dimensions, data quality dimensions, etc. Data quality monitoring and control can help to identify and prevent potential data quality problems, as well as to evaluate the effectiveness of data quality improvement actions.
This service involves establishing and enforcing the policies, roles, and responsibilities for data quality management, as well as ensuring compliance with data quality standards and regulations.
Those are the main services we offer in regards to data quality management.
Evaluating data through diverse metrics and techniques to spot errors, gauge their impact on business processes, and enact corrections.
Cleaning, standardizing, matching and transforming data to correct typos, identify and filling missing values, correct inconsistencies, homogenizing values and formats and other similar tasks.
Comparing data points from multiple sources to identify records that refer to the same real-world entity. By comparing data points like names, addresses, or IDs, data matching will create a more complete picture about your business
Designing a comprehensive Data Quality Policy and create a quality manual to include principles and objects as well as processes and detailed guidelines. This can be integrated in the existing quality system or certification (such as ISO 9001) or lead to a more specialized certification like ISO 8000.
It is frequently necessary to reconfigure a system to address quality issues. It is usually about setting up enforcement rules or facilitate control mechanisms.
A thorough and objective assessment of a company's data quality with the purpose to be presented to a third party with a legitimate interest to evaluate this part of the business.
The quantity and quality of data are both crucial factors that determine the value and accuracy of data analysis and decision making. Having a large quantity of data can increase the statistical power and reduce the sampling error of data analysis. It is certain that data quantity is also important, either for the purposes of a traditional analysis or for an AI enabled process. There is always a minimum of data needed, to be able to help business and organizations. Quantity is usually perceived as complementary to quality. Nevertheless, there are cases where quantity can be conceived as directly related to quality in different ways. There are certainly many examples where the quest for large amounts of data may result in the drop of quality. In the other hand, an increase of quantity may be the enabler to achieve specific quality standards, mainly related to relevance and consistency. Furthermore, having more data gives always the option to dismiss a part of them that will consider of insufficient quality.
You can find below some related articles from our blog
A modern CRM system serves as the central nervous system for managing interactions and data related...
The Power of Organization for the Solopreneur Juggling client emails, chasing deadlines, and...
There is a common experience of people who have been working with CRMs for a long time: the problem...